Martin Gölz runner-up in Three-Minute-Thesis competition at EUSIPCO 2023
2023/09/18
At this year's European Signal Processing Conference (EUSIPCO) in Helsinki, Finland, Martin Gölz finished second in the Three Minute Thesis Contest with the presentation of his PhD topic “Spatial Inference via Multiple Hypothesis Testing”.
The competition is held annually during organized by the European Association for Signal Processing (EURASIP) and has over the years developed into a highly anticipated event. This year's edition saw a record number of 56 participants. Each competitor submitted a 3-minute video, based on which the best eleven participants received an invitation to participate in the final in Helsinki. There, the candidates from different universities had to present their doctoral topic live in at most three minutes in front of more than 100 spectators. The audience then voted to select the three winners. EUSIPCO
has been researching anomaly detection in spatial signals via wireless sensor networks since April 2019. A spatial signal is any physical phenomenon that varies as a function of location, such as air quality or wireless signal strength. Using multiple hypothesis testing, the areas where a spatial signal behaves differently from a well-defined baseline state can be identified under statistical error guarantees. This is useful, for instance, in autonomous driving, where self-driving vehicles should be aware of streets with lacking wireless coverage to avoid losing signal while driving. Martin Gölz
The doctoral work of Martin Gölz is supervised by Prof. Dr.-Ing. Abdelhak Zoubir and from Aalto University, Finland. Prof. D.Sc. (EE) Visa Koivunen
Congratulations, Martin!
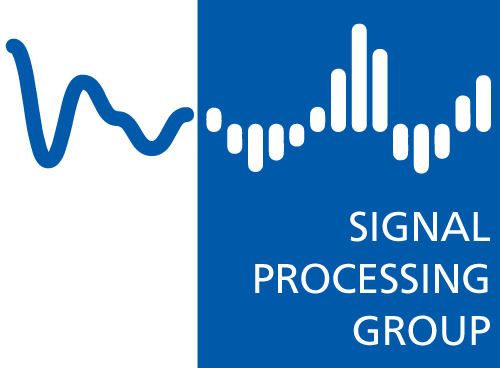