Ann-Kathrin Seifert wins Three Minute Thesis competition at EUSIPCO 2019
2019/09/18
At this year's European Signal Processing Conference (EUSIPCO) in A Coruña, Spain, Ann-Kathrin Seifert won the Three Minute Thesis Contest by presenting her PhD topic “Signal Processing for Radar-based Medical Gait Analysis”.
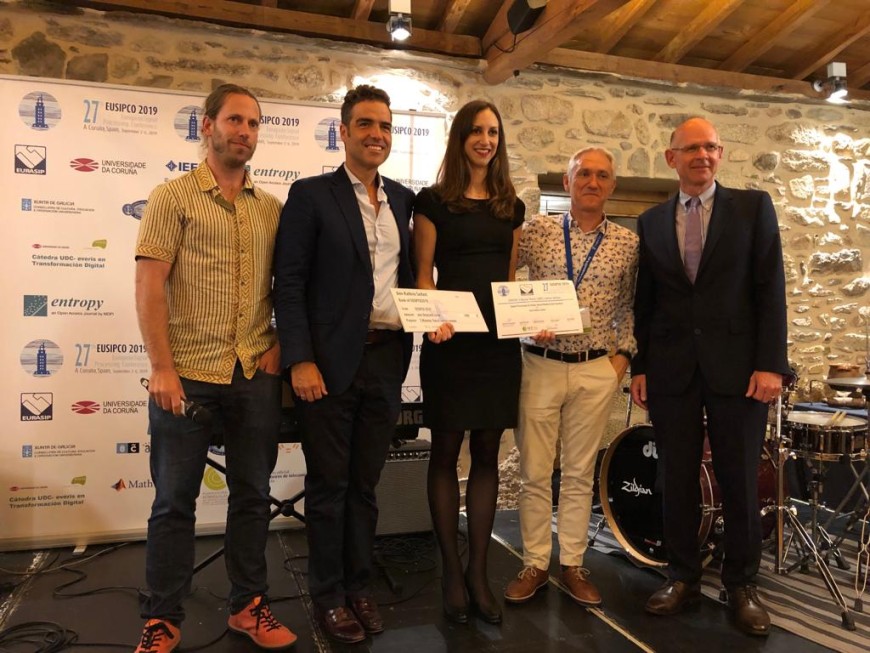
The competition, held for the fifth consecutive time at , is organised annually by the European Association for Signal Processing (EURASIP). This year, ten doctoral candidates were invited to EUSIPCO in A Coruña on the basis of 3-minute videos submitted in advance. The candidates from different universities had to present their doctoral topic live in three minutes in front of 125 spectators. The audience then voted to select the three winners. EUSIPCO
has been researching radar-based gait detection since October 2015. Using the so-called radar micro-Doppler signatures, abnormalities in the gait can be detected without the need for wearable sensors. The doctoral work of Ann-Kathrin Seifert is supervised by Prof. Dr.-Ing. Abdelhak Zoubir and Ann-Kathrin Seifert from Villanova University, PA, USA. Prof. Dr. Moeness Amin
Congratulations, Ann-Kathrin!
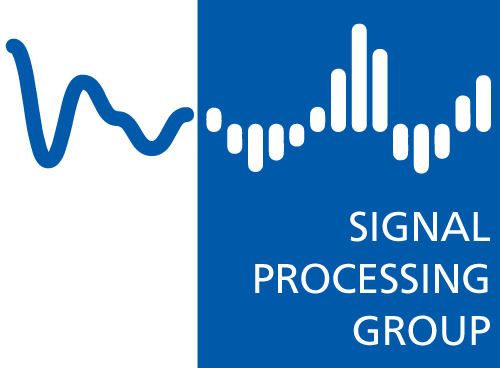