Michael Leigsnering, Fauzia Ahmad, Moeness Amin, und Abdelhak Zoubir mit dem 2014 M. Barry Carlton Award ausgezeichnet
Beitrag wurde als „Best Paper“ der IEEE Transactions on Aerospace and Electronic Systems des Jahres 2014 beurteilt
28.08.2019
Der Artikel von Michael Leigsnering, Fauzia Ahmad, Moeness Amin, und Abdelhak Zoubir mit dem Titel “Multipath Exploitation in Through-the-Wall Radar Imaging Using Sparse Reconstruction” erschien im April 2014 in der Ausgabe der IEEE Transactions on Aerospace and Electronic Systems.
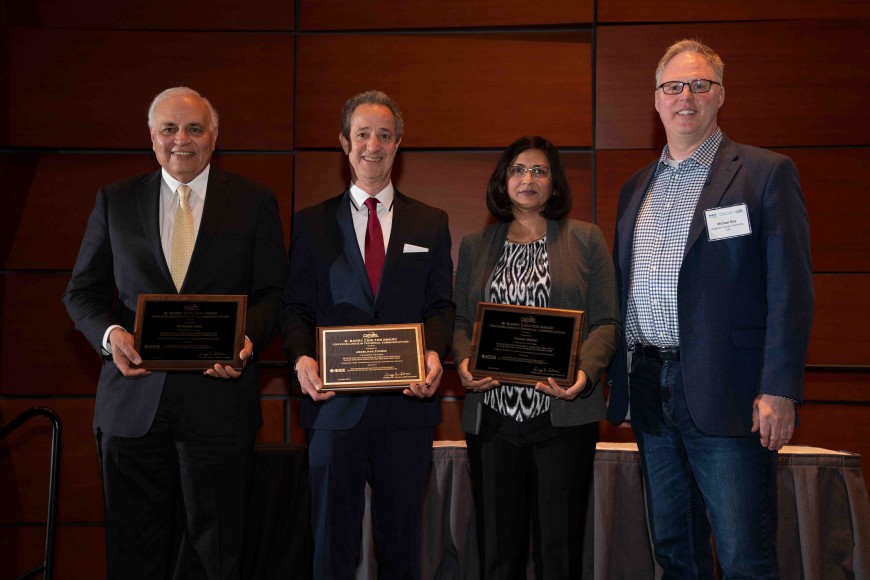
Michael Leigsnering ist ehemaliger Mitarbeiter des Fachgebiets Signalverarbeitung und wurde 2015 promoviert. Seine Forschungsarbeit zum Thema „Through-the-wall Imaging“ entstand aus der anhaltenden Kooperation mit dem . Center for Advanced Communcations, Villanova University, PA, USA
Der ist einer der ältesten IEEE Auszeichnungen und wird für herausragende Artikel in den M. Barry Carlton Preis verliehen. Der Preis wurde den Authoren auf der IEEE Radar Conference in Boston überreicht. IEEE Transactions on Aerospace and Electronic Systems
Herzlichen Glückwunsch an alle Authoren!
Kurzzusammenfassung des Artikels:
Multipath exploitation and compressive sensing (CS) have both been applied independently to through-the-wall radar imaging (TWRI). Fast and efficient data acquisition is desired in scenarios where multipath effects cannot be neglected. Hence, we combine the two methods to achieve good image reconstruction in multipath environments from few spatial and frequency measurements. Ghost targets appear in the scene primarily due to specular reflections from interior walls and multiple reflections within the front wall. Assuming knowledge of the room geometry, we can invert the multipath model and eliminate ghosts by means of CS. We develop effective methods for the reconstruction of stationary scenes, which employ a group sparse CS approach. Additionally, we separate the target and wall contributions to the image by a sparse reconstruction approach joining wall and target models, which allows suppression of the ghosts and increased signal-to-clutter ratio (SCR) at the target locations. Effectiveness of the proposed approach is demonstrated using both simulated and real data.
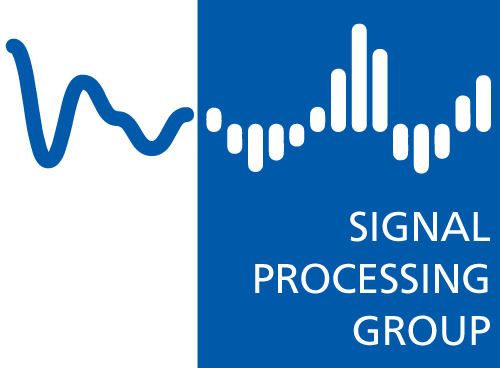