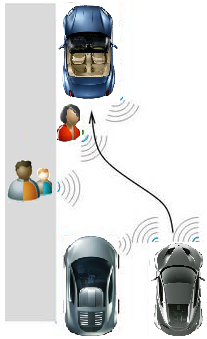
According to the World Health Organization, over 1.2 million people die in road traffic accidents every year and approximately additional 20-50 million sustain injuries of different degrees. In fact, the majority of accidents is caused by mistakes committed by the human driver.
Many existing driver assistance systems such as Anti-Block-System (ABS) and Electronic Stability Program (ESP) provide basic assistance for the control of the vehicle in critical situations. Thus, these systems play a crucial role in modern cars, protecting the driver and the passengers.
Due to the increasing complexity of traffic, we seek for advanced driver assistance systems (ADAS) that are able to act according to the environment of the vehicle. Typically, ADAS require the evaluation of huge amounts of data captured by different sensor systems, involving radar, ultrasonic, video, lidar etc. Consequently, efficient and adaptive Signal Processing combined with Machine Learning are key elements for the success of those systems.
The Signal Processing Group is collaborating with the leading automotive industry, e.g. Adam Opel AG and Continental GmbH, which provide expertise knowledge and real data experiments for automotive applications. Research for automotive applications at the SPG involves
- Regression and Classification
- Decision Making
- Radar Signal Processing
- Detection
- Data Fusion
For more information on Automotive Applications, see the sections below or contact the respective Research Associates.