Research Areas
The main theoretical foundations are:
- Signal Detection and Classification
- Robust Statistics for Signal Processing
- Parameter Estimation and Signal Reconstruction
Signal Detection and Classification
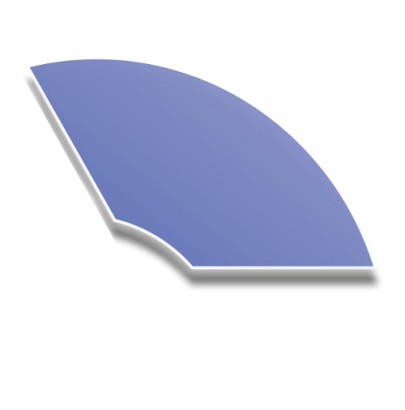
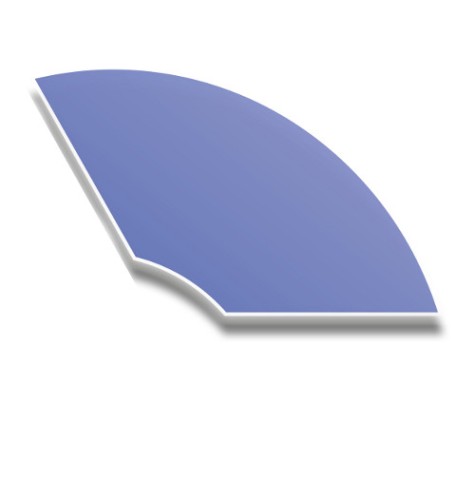
Detection and Classification are fundamental tasks in signal processing. The decision making problem underpinning both is as follows: Given a finite number of possible hypotheses or scenarios, decide for the one that is most likely to be true. This task can either be solved using statistical models that are compared to the observed data, or by means of training data that is used to learn about characteristic features of the observations.
Examples for detection and classification problems are ubiquitous: Does a mobile phone receive a signal form a base station? Is a person on a picture a man or a woman? Is the blood pressure of a patient critically low or high? How many people are left in a building that needs to be evacuated? Signal Processing helps answering all of these questions.
In detection, the focus of the signal processing group is on robust, sequential and distributed methods. In classification, the emphasis is on Bayesian decision theory and bio-inspired methods. The overall goal is to design techniques that are widely applicable, yet rooted in mathematical statistics with well defined properties and performance bounds.
Parameter Estimation and Signal Reconstuction
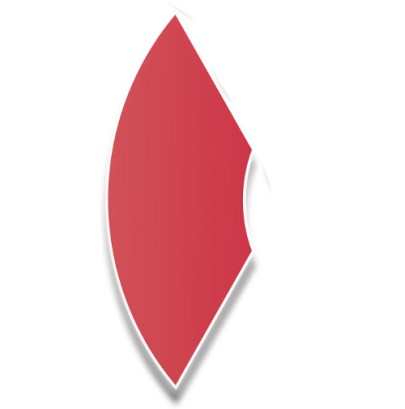
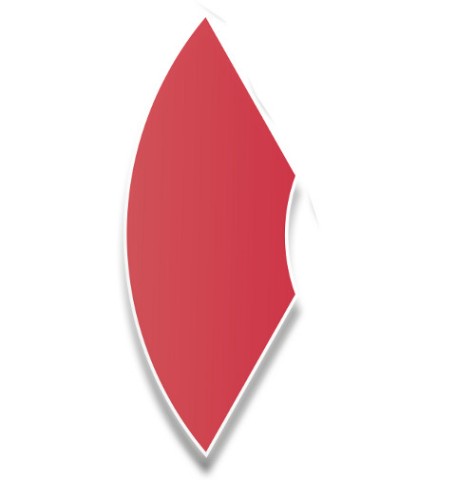
Learning about the behavior of economic phenomena, measuring the heart rate of a fetus, determining the position of an aircraft or an underwater object, and reconstructing a 3D image from a set of radar measurements are all examples of applications, where parameter estimation and signal reconstruction are needed to infer meaningful information from measured data.
Often, we measure data to extract a specific piece of information that cannot be measured directly, as it is hidden in noise or not directly attainable. This is where parameter estimation comes into play: by building a suitable statistical model that incorporates parameters describing the behavior of the system under test, it becomes possible to better understand and describe the system. In signal reconstruction, we want to use the measurements to restore the signal of interest. The signal processing group aims at developing robust, as well as distributed parameter estimation methods. The focus in signal reconstruction is on sparse, robust techniques.
Robust Statistics for Signal Processing
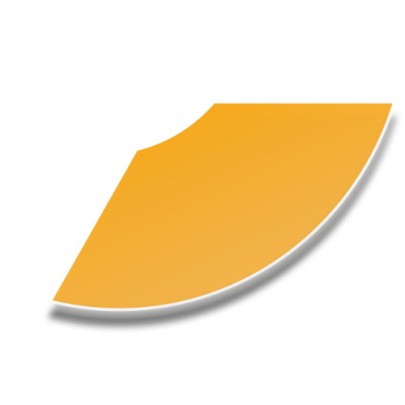
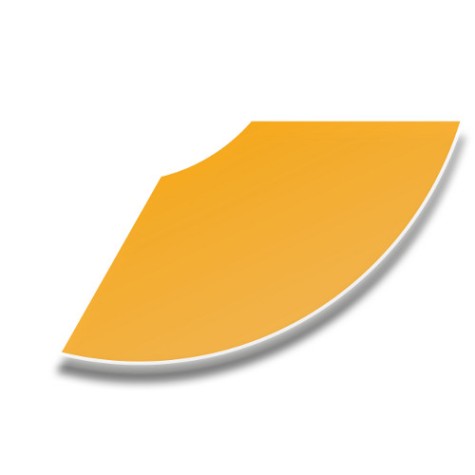
In many areas of engineering, we encounter measurements that don’t follow the normal distribution, and in fact the distribution of the measurements is far from Gaussian. This can be due to the presence of outliers, which cause the distribution to be heavy-tailed or simply due to nonlinear processing encountered in many practical systems. Classical statistical signal processing, however, often relies on strong assumptions, i.e. optimal estimators and detectors are derived based e.g. on a probability distribution of the sensor noise (typically the normal distribution). Optimality, however, is only achieved when the underlying assumptions hold and the performance of optimal procedures may deteriorate significantly, even for minor departures from the assumed model. These situations enforce the need for robust signal processing methods, which are close-to optimal in nominal conditions and highly reliable for real-life data, even if the assumptions are only approximately valid. In engineering, robust estimators and detectors have been of interest since the early days of digital signal processing.